Why this course?
This course will equip you with the specialist knowledge and technical skills you need to effectively apply the powerful tools and insights of data science to your work.
Why specialise in data science?
Key benefits:
-
Tutor-led - University of Southampton academics will guide you through the content and answer your questions, providing the support you need. Read Head of Learning Manuel León-Urrutia's blog post for more on the benefits of tutor-led courses.
-
Continuing Professional Development (CPD) accredited - this will help you demonstrate your commitment to upskilling at your next work appraisal.
-
Hands-on - learn how to apply AI capabilities within your own workplace.
-
Developed by our pioneering Data Science team - the University of Southampton is ranked among the top 100 universities globally.
Who is this course for?
In today’s data-driven economy, an organisation’s success or failure can rest on its ability to leverage insights and develop business critical analytics.
This 6-week part-time, online course is designed to introduce you to the theoretical components of data science and give you hands-on experience in producing solutions to data science problems using Python.
This course will build on your existing data skills and arm you with the practical capabilities to deliver an immediate impact on day-to-day business. You’ll take a hands-on approach to learning data skills through interactive exercises that will offer more exposure to Python and allow you to try out many of the techniques and concepts covered in the taught material.
With our support, you will extend your knowledge and expertise across data mining, machine-learning, and data visualisation to become a highly-skilled data scientist.
How can tutor-led courses set you up for success?
Head of Learning Manuel León-Urrutia explains how tutoring can help you apply data science skills more effectively in our blog post:
Discover more >
Can data science training help you get a job in the field?
Watch our video to hear Head of Learning Manuel León-Urrutia explain what companies are looking for:
What's it like to study the Fundamentals of Data Science (Technical) course?
Watch our short video to hear about students' experience:
Why choose data science training with the Academy?
Discover the benefits of studying data science online, including how our training can be applied to your work. Watch Head of Learning Manuel León-Urrutia answer key questions about the Academy's short courses:
What you'll learn
The course runs over 6 weeks and is broken down into manageable weekly topics:
Week 1: Welcome and course information
-
Welcome and introduction to the course
-
What data science is and why it’s important
-
A ‘hands-on’ Jupyter familiarisation activity
-
Python Primer
-
Glossary of terminology
Week 2: Introduction to core concepts and technologies
-
The data science process
-
A data science toolkit
-
Types of data and example applications
Week 3: Data collection and management
-
Sources of data
-
Data collection and APIs
-
Exploring and fixing data
-
Data storage and management
-
Using multiple data sources
Week 4: Data analysis
-
Introduction to statistics
-
Basic machine-learning algorithms
Week 5: Data visualisation
-
Types of data visualisation
-
Data for visualisation
-
Technologies for visualisation
Week 6: Future of data science
-
An exploration of the future of data science
After successfully completing the course, you’ll be able to:
-
Understand key concepts in data science and their real-world applications
-
Explain how data is collected, managed and stored in the context of data science
-
Implement data collection and management scripts using MongoDB
-
Demonstrate an understanding of statistics and machine-learning concepts vital for data science
-
Produce Python code to statistically analyse a dataset
-
Plan and generate visualisations from data using tools such as Python and Bokeh
-
Work effectively with live data and utilise the opportunities presented by cloud services
How you'll learn
Guidance thoughout the course
A key benefit of choosing Southampton Data Science Academy over some of the other online courses available is that our courses are tutor-led. A tutor is just as important with an online course as it is in a physical classroom. A good tutor’s passion for the subject will motivate you and inspire you, making the content stick in your mind.
Our tutors are data science experts who can make complex ideas accessible. If you don’t understand the course material right away they can provide an alternative explanation or use a different example. If you have a question about the content, our tutors are available to answer it. They will work with you to make sure you understand the subject fully and are on track to complete the course successfully.
How can tutor-led courses set you up for success?
Head of Learning Manuel León-Urrutia explains how tutoring can help you apply data science skills more effectively in our blog post:
Discover more >
Hands-on learning that you can immediately apply to your work
Learning is hands-on, using real-life business examples to demonstrate how you can immediately harness and apply the power of data science to your work. Our online learning platform is easy to access via smartphone, tablet or desktop – anytime and from anywhere in the world. You’ll join a global online network of like-minded professionals and take part in group discussions, Q&A sessions and video tutorials.
Assessment methods and feedback
You'll complete three assignments, worth 33% of your final mark each. Your online tutor will provide feedback at the end of the course, consisting of marks and comments on each assignment. After passing the course, you'll receive a downloadable electronic certificate.
Find out more about how you'll learn with Southampton Data Science Academy.
CPD-accredited
Continuing Professional Development, or CPD, describes the efforts made by professionals to develop their skillsets. With the CPD Certification Service, courses and training offered by institutions across the globe are assessed to ensure they are executed with proficiency and integrity.
This course is CPD-accredited. This means your education with us meets benchmarks recognised throughout the industry, internationally.
Many employers will ask for evidence at appraisals of the impact CPD has had on your career. A CPD-accredited Southampton Data Science course is a great way to demonstrate your commitment to upskilling, especially given that you learn practical techniques and tools that can be immediately applied to your professional life.
Some of the real-world benefits provided by our CPD-accredited courses include:
-
The ability to stay current and competitive with your industry expertise
-
Support maintaining the professional standard of your qualifications and registrations
-
Training to build confidence in new skills and your overall capabilities
-
Insights to improve your career trajectory and preserve job security
-
The possibility of exploring new progression paths and widening your scope
-
The adaptability to handle change across teams, organisations, and fields
Discover more of the career benefits of taking CPD-accredited training courses:
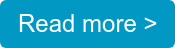
Your online tutors
Visit our Tutors page to find out more about the expert tutors who will guide you through our short, online courses:
Discover more >